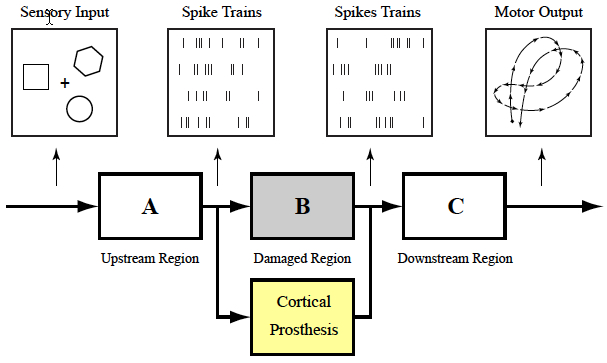
Cortical memory prosthesis uses internal brain signals (e.g., spike
trains) as inputs and outputs, bypassing damaged region (Dong Song et al.)
A brain prosthesis designed to help individuals suffering from memory loss has been developed by researchers at USC and Wake Forest Baptist Medical Center.
The prosthesis, which uses a small array of electrodes implanted into the brain, has performed well in laboratory testing in animals and is currently being evaluated in human patients.
The device builds on decades of research by Ted Berger and relies on a new algorithm created by Dong Song, both of the USC Viterbi School of Engineering. The development also builds on more than a decade of collaboration with Sam Deadwyler and Robert Hampson of the Department of Physiology & Pharmacology of Wake Forest Baptist, who have collected the neural data used to construct the models and algorithms.

Electrode array for monitoring and duplicating hippocampus neuron activity (credit: T. Berger et al./Journal of Neural Engineering)
When your brain receives a sensory input, it creates a memory in the form of a complex electrical signal that travels through multiple regions of the hippocampus, the memory center of the brain. At each region, the signal is re-encoded until it reaches the final region as a wholly different signal that is sent off for long-term storage.
If there’s damage at any region that prevents this translation, there is the possibility that long-term memory will not be formed. That’s why an individual with hippocampal damage (for example, due to Alzheimer’s disease) can recall events from a long time ago — things that were already translated into long-term memories before the brain damage occurred — but have difficulty forming new long-term memories.
Bypassing a damaged hippocampal section
Song and Berger found a way to accurately mimic how a memory is translated from short-term memory into long-term memory, using data obtained by Deadwyler and Hampson, first from animals, and then from humans. Their prosthesis is designed to bypass a damaged hippocampal section and provide the next region with the correctly translated memory.
That’s despite the fact that there is currently no way of “reading” a memory just by looking at its electrical signal. “It’s like being able to translate from Spanish to French without being able to understand either language,” Berger said.
Their research was presented at the 37th Annual International Conference of the IEEE Engineering in Medicine and Biology Society in Milan on August 27, 2015.
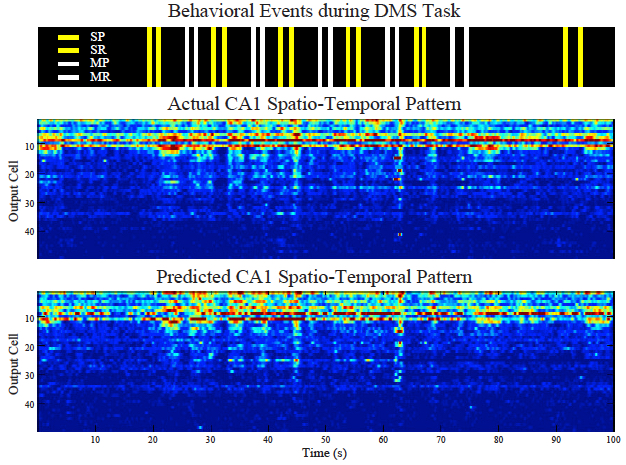
Predicting CA1 spatio-temporal patterns from CA3 spatio-temporal patterns with the sparse MIMO model. SP: sample presentation; SR: sample response; MP: match presentation; MR: match response. (Dong Song et al.)
The effectiveness of the model was tested by the USC and Wake Forest Baptist teams. With the permission of patients who had electrodes implanted in their hippocampi to treat chronic seizures, Hampson and Deadwyler read the electrical signals created during memory formation at two regions of the hippocampus, then sent that information to Song and Berger to construct the model.
The team then fed those signals into the model and read how the signals generated from the first region of the hippocampus were translated into signals generated by the second region of the hippocampus.
In hundreds of trials conducted with nine patients, the algorithm accurately predicted how the signals would be translated with about 90 percent accuracy.
“Being able to predict neural signals with the USC model suggests that it can be used to design a device to support or replace the function of a damaged part of the brain,” Hampson said.
Next, to try to bypass the damage and enable the formation of an accurate long-term memory, the team will attempt to send the translated signal back into the brain of a patient with damage at one of the regions.
Abstract of Sparse Generalized Volterra Model of Human Hippocampal Spike Train Transformation for Memory Prostheses
In order to build hippocampal prostheses for restoring memory functions, we build multi-input, multi-output (MIMO) nonlinear dynamical models of the human hippocampus. Spike trains are recorded from the hippocampal CA3 and CA1 regions of epileptic patients performing a memory-dependent delayed match-to-sample task. Using CA3 and CA1 spike trains as inputs and outputs respectively, second-order sparse generalized Laguerre-Volterra models are estimated with group lasso and local coordinate descent methods to capture the nonlinear dynamics underlying the spike train transformations. These models can accurately predict the CA1 spike trains based on the ongoing CA3 spike trains and thus will serve as the computational basis of the hippocampal memory prosthesis.