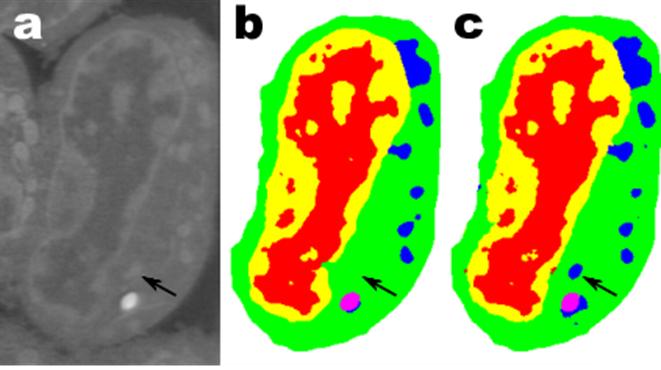
(a) Raw microscopy image of a slice of mouse lymphblastoid cells. (b) Reconstructed image using time-consuming manual segmentation — note missing data (arrow). (c) Equivalent output of the new “Mixed-Scale Dense Convolution Neural Network” algorithm with 100 layers. (credit: Data from A. Ekman and C. Larabell, National Center for X-ray Tomography.)
Mathematicians at Lawrence Berkeley National Laboratory (Berkeley Lab) have developed a radical new approach to machine learning: a new type of highly efficient “deep convolutional neural network” that can automatically analyze complex experimental scientific images from limited data.*
As experimental facilities generate higher-resolution images at higher speeds, scientists struggle to manage and analyze the resulting data, which is often done painstakingly by hand.
For example, biologists record cell images and painstakingly outline the borders and structure by hand. One person may spend weeks coming up with a single fully three-dimensional image of a cellular structure. Or materials scientists use tomographic reconstruction to peer inside rocks and materials, and then manually label different regions, identifying cracks, fractures, and voids by hand. Contrasts between different yet important structures are often very small and “noise” in the data can mask features and confuse the best of algorithms.
To meet this challenge, mathematicians Daniël Pelt and James Sethian at Berkeley Lab’s Center for Advanced Mathematics for Energy Research Applications (CAMERA)** attacked the problem of machine learning from very limited amounts of data — to do “more with less.”
Their goal was to figure out how to build an efficient set of mathematical “operators” that could greatly reduce the number of required parameters.
“Mixed-Scale Dense” network learns quickly with far fewer images
Many applications of machine learning to imaging problems use deep convolutional neural networks (DCNNs), in which the input image and intermediate images are convolved in a large number of successive layers, allowing the network to learn highly nonlinear features. To train deeper and more powerful networks, additional layer types and connections are often required. DCNNs typically use a large number of intermediate images and trainable parameters, often more than 100 million, to achieve results for difficult problems.
The new method the mathematicians developed, “Mixed-Scale Dense Convolution Neural Network” (MS-D), avoids many of these complications. It “learns” much more quickly than manually analyzing the tens or hundreds of thousands of labeled images required by typical machine-learning methods, and requires far fewer images, according to Pelt and Sethian.
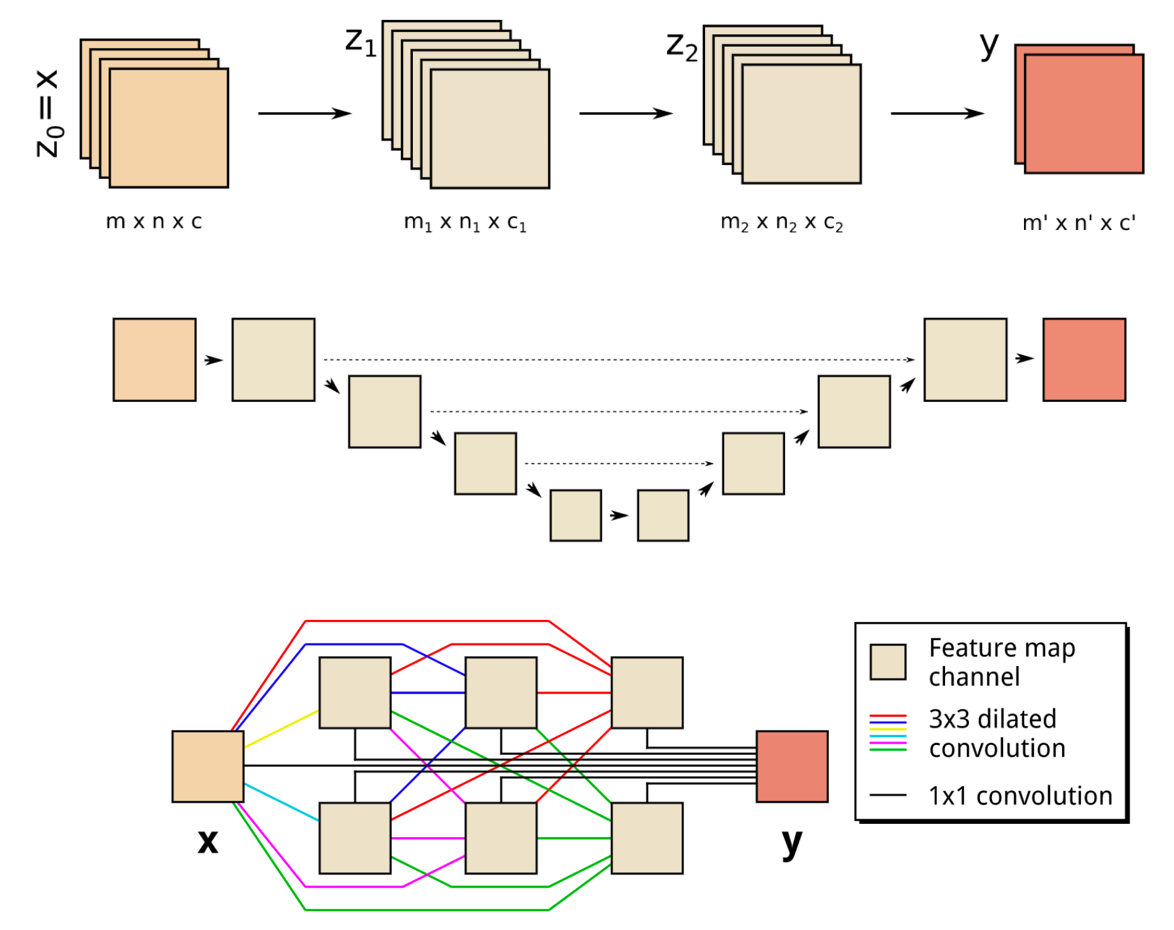
(Top) A schematic representation of a two-layer CNN architecture. (Middle) A schematic representation of a common DCNN architecture with scaling operations; downward arrows represent downscaling operations, upward arrows represent upscaling operations and dashed arrows represent skipped connections. (Bottom) Schematic representation of an MS-D network; colored lines represent 3×3 dilated convolutions, with each color corresponding to a different dilation. (credit: Daniël Pelt and James Sethian/PNAS, composited by KurzweilAI)
The “Mixed-Scale Dense” network architecture calculates ”dilated convolutions” — a substitute for complex scaling operations. To capture features at various spatial ranges, it employs multiple scales within a single layer, and densely connects all intermediate images. The new algorithm achieves accurate results with few intermediate images and parameters, eliminating both the need to tune hyperparameters and additional layers or connections to enable training, according to the researchers.***
“In many scientific applications, tremendous manual labor is required to annotate and tag images — it can take weeks to produce a handful of carefully delineated images,” said Sethian, who is also a mathematics professor at the University of California, Berkeley. “Our goal was to develop a technique that learns from a very small data set.”
Details of the algorithm were published Dec. 26, 2017 in a paper in the Proceedings of the National Academy of Sciences.
Radically transforming our ability to understand disease
The MS-D approach is already being used to extract biological structure from cell images, and is expected to provide a major new computational tool to analyze data across a wide range of research areas. In one project, the MS-D method needed data from only seven cells to determine the cell structure.
“The breakthrough resulted from realizing that the usual downscaling and upscaling that capture features at various image scales could be replaced by mathematical convolutions handling multiple scales within a single layer,” said Pelt, who is also a member of the Computational Imaging Group at the Centrum Wiskunde & Informatica, the national research institute for mathematics and computer science in the Netherlands.
“In our laboratory, we are working to understand how cell structure and morphology influences or controls cell behavior. We spend countless hours hand-segmenting cells in order to extract structure, and identify, for example, differences between healthy vs. diseased cells,” said Carolyn Larabell, Director of the National Center for X-ray Tomography and Professor at the University of California San Francisco School of Medicine.
“This new approach has the potential to radically transform our ability to understand disease, and is a key tool in our new Chan-Zuckerberg-sponsored project to establish a Human Cell Atlas, a global collaboration to map and characterize all cells in a healthy human body.”
To make the algorithm accessible to a wide set of researchers, a Berkeley team built a web portal, “Segmenting Labeled Image Data Engine (SlideCAM),” as part of the CAMERA suite of tools for DOE experimental facilities.
High-resolution science from low-resolution data
A different challenge is to produce high-resolution images from low-resolution input. If you’ve ever tried to enlarge a small photo and found it only gets worse as it gets bigger, this may sound close to impossible.
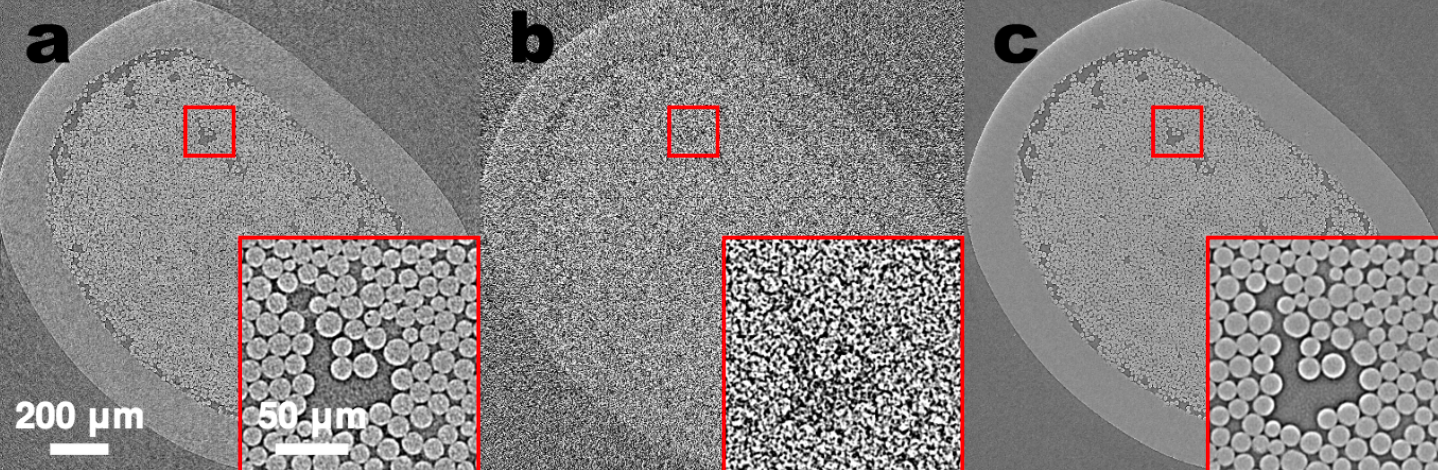
(a) Tomographic images of a fiber-reinforced mini-composite, reconstructed using 1024 projections. Noisy images (b) of the same object were obtained by reconstructing using only 128 projections, and were used as input to an MS-D network (c). A small region indicated by a red square is shown enlarged in the bottom-right corner of each image. (credit: Daniël Pelt and James A. Sethian/PNAS)
As an example, imagine trying to de-noise tomographic reconstructions of a fiber-reinforced mini-composite material. In an experiment described in the paper, images were reconstructed using 1,024 acquired X-ray projections to obtain images with relatively low amounts of noise. Noisy images of the same object were then obtained by reconstructing using only 128 projections. Training inputs to the Mixed-Scale Dense network were the noisy images, with corresponding noiseless images used as target output during training. The trained network was then able to effectively take noisy input data and reconstruct higher resolution images.
Pelt and Sethian are now applying their approach to other new areas, such as real-time analysis of images coming out of synchrotron light sources, biological reconstruction of cells, and brain mapping.
* Inspired by the brain, convolutional neural networks are computer algorithms that have been successfully used in analyzing visual imagery. “Deep convolutional neural networks (DCNNs) use a network architecture similar to standard convolutional neural networks, but consist of a larger number of layers, which enables them to model more complicated functions. In addition, DCNNs often include downscaling and upscaling operations between layers, decreasing and increasing the dimensions of feature maps to capture features at different image scales.” — Daniël Pelt and James A. Sethian/PNAS
** In 2014, Sethian established CAMERA at the Department of Energy’s (DOE) Lawrence Berkeley National Laboratory as an integrated, cross-disciplinary center to develop and deliver fundamental new mathematics required to capitalize on experimental investigations at DOE Office of Science user facilities. CAMERA is part of the lab’s Computational Research Division. It is supported by the offices of Advanced Scientific Computing Research and Basic Energy Sciences in the Department of Energy’s Office of Science. The single largest supporter of basic research in the physical sciences in the United States, the Office of Science is working to address some of the most pressing challenges of our time.
*** “By combining dilated convolutions and dense connections, the MS-D network architecture can achieve accurate results with significantly fewer feature maps and trainable parameters than existing architectures, enabling accurate training with relatively small training sets. MS-D networks are able to automatically adapt by learning which combination of dilations to use, allowing identical MS-D networks to be applied to a wide range of different problems.” — Daniël Pelt and James A. Sethian/PNAS
Abstract of A mixed-scale dense convolutional neural network for image analysis
Deep convolutional neural networks have been successfully applied to many image-processing problems in recent works. Popular network architectures often add additional operations and connections to the standard architecture to enable training deeper networks. To achieve accurate results in practice, a large number of trainable parameters are often required. Here, we introduce a network architecture based on using dilated convolutions to capture features at different image scales and densely connecting all feature maps with each other. The resulting architecture is able to achieve accurate results with relatively few parameters and consists of a single set of operations, making it easier to implement, train, and apply in practice, and automatically adapts to different problems. We compare results of the proposed network architecture with popular existing architectures for several segmentation problems, showing that the proposed architecture is able to achieve accurate results with fewer parameters, with a reduced risk of overfitting the training data.