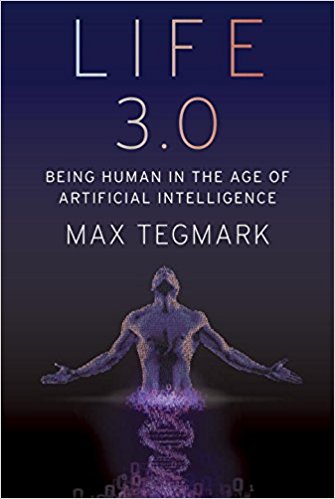
In his new book Life 3.0: Being Human in the Age of Artificial Intelligence, MIT physicist and AI researcher Max Tegmark explores the future of technology, life, and intelligence.
The question of how to define life is notoriously controversial. Competing definitions abound, some of which include highly specific requirements such as being composed of cells, which might disqualify both future intelligent machines and extraterrestrial civilizations. Since we don’t want to limit our thinking about the future of life to the species we’ve encountered so far, let’s instead define life very broadly, simply as a process that can retain its complexity and replicate.
What’s replicated isn’t matter (made of atoms) but information (made of bits) specifying how the atoms are arranged. When a bacterium makes a copy of its DNA, no new atoms are created, but a new set of atoms are arranged in the same pattern as the original, thereby copying the information.
In other words, we can think of life as a self-replicating information-processing system whose information (software) determines both its behavior and the blueprints for its hardware.
Like our Universe itself, life gradually grew more complex and interesting, and as I’ll now explain, I find it helpful to classify life forms into three levels of sophistication: Life 1.0, 2.0 and 3.0.
It’s still an open question how, when and where life first appeared in our Universe, but there is strong evidence that here on Earth life first appeared about 4 billion years ago.
Before long, our planet was teeming with a diverse panoply of life forms. The most successful ones, which soon outcompeted the rest, were able to react to their environment in some way.
Specifically, they were what computer scientists call “intelligent agents”: entities that collect information about their environment from sensors and then process this information to decide how to act back on their environment. This can include highly complex information processing, such as when you use information from your eyes and ears to decide what to say in a conversation. But it can also involve hardware and software that’s quite simple.
For example, many bacteria have a sensor measuring the sugar concentration in the liquid around them and can swim using propeller-shaped structures called flagella. The hardware linking the sensor to the flagella might implement the following simple but useful algorithm: “If my sugar concentration sensor reports a lower value than a couple of seconds ago, then reverse the rotation of my flagella so that I change direction.”
You’ve learned how to speak and countless other skills. Bacteria, on the other hand, aren’t great learners. Their DNA specifies not only the design of their hardware, such as sugar sensors and flagella, but also the design of their software. They never learn to swim toward sugar; instead, that algorithm was hard- coded into their DNA from the start.
There was of course a learning process of sorts, but it didn’t take place during the lifetime of that particular bacterium. Rather, it occurred during the preceding evolution of that species of bacteria, through a slow trial-and-error process spanning many generations, where natural selection favored those random DNA mutations that improved sugar consumption. Some of these mutations helped by improving the design of flagella and other hardware, while other mutations improved the bacterial information-processing system that implements the sugar-finding algorithm and other software.
“Tegmark’s new book is a deeply thoughtful guide to the most important conversation of our time, about how to create a benevolent future civilization as we merge our biological thinking with an even greater intelligence of our own creation.” — Ray Kurzweil, Inventor, Author and Futurist, author of The Singularity Is Near and How to Create a Mind
Such bacteria are an example of what I’ll call “Life 1.0”: life where both the hardware and software are evolved rather than designed. You and I, on the other hand, are examples of “Life 2.0”: life whose hardware is evolved, but whose software is largely designed. By your software, I mean all the algorithms and knowledge that you use to process the information from your senses and decide what to do—everything from the ability to recognize your friends when you see them to your ability to walk, read, write, calculate, sing and tell jokes.
You weren’t able to perform any of those tasks when you were born, so all this software got programmed into your brain later through the process we call learning. Whereas your childhood curriculum is largely designed by your family and teachers, who decide what you should learn, you gradually gain more power to design your own software.
Perhaps your school allows you to select a foreign language: Do you want to install a software module into your brain that enables you to speak French, or one that enables you to speak Spanish? Do you want to learn to play tennis or chess? Do you want to study to become a chef, a lawyer or a pharmacist? Do you want to learn more about artificial intelligence (AI) and the future of life by reading a book about it?
This ability of Life 2.0 to design its software enables it to be much smarter than Life 1.0. High intelligence requires both lots of hardware (made of atoms) and lots of software (made of bits). The fact that most of our human hardware is added after birth (through growth) is useful, since our ultimate size isn’t limited by the width of our mom’s birth canal. In the same way, the fact that most of our human software is added after birth (through learning) is useful, since our ultimate intelligence isn’t limited by how much information can be transmitted to us at conception via our DNA, 1.0-style.
I weigh about twenty-five times more than when I was born, and the synaptic connections that link the neurons in my brain can store about a hundred thousand times more information than the DNA that I was born with. Your synapses store all your knowledge and skills as roughly 100 terabytes’ worth of information, while your DNA stores merely about a gigabyte, barely enough to store a single movie download. So it’s physically impossible for an infant to be born speaking perfect English and ready to ace her college entrance exams: there’s no way the information could have been preloaded into her brain, since the main information module she got from her parents (her DNA) lacks sufficient information-storage capacity.
The ability to design its software enables Life 2.0 to be not only smarter than Life 1.0, but also more flexible. If the environment changes, 1.0 can only adapt by slowly evolving over many generations. Life 2.0, on the other hand, can adapt almost instantly, via a software update. For example, bacteria frequently encountering antibiotics may evolve drug resistance over many generations, but an individual bacterium won’t change its behavior at all; in contrast, a girl learning that she has a peanut allergy will immediately change her behavior to start avoiding peanuts.
This flexibility gives Life 2.0 an even greater edge at the population level: even though the information in our human DNA hasn’t evolved dramatically over the past fifty thousand years, the information collectively stored in our brains, books and computers has exploded. By installing a software module enabling us to communicate through sophisticated spoken language, we ensured that the most useful information stored in one person’s brain could get copied to other brains, potentially surviving even after the original brain died.
By installing a software module enabling us to read and write, we became able to store and share vastly more information than people could memorize. By developing brain software capable of producing technology (i.e., by studying science and engineering), we enabled much of the world’s information to be accessed by many of the world’s humans with just a few clicks.
This flexibility has enabled Life 2.0 to dominate Earth. Freed from its genetic shackles, humanity’s combined knowledge has kept growing at an accelerating pace as each breakthrough enabled the next: language, writing, the printing press, modern science, computers, the internet, etc. This ever-faster cultural evolution of our shared software has emerged as the dominant force shaping our human future, rendering our glacially slow biological evolution almost irrelevant.
Yet despite the most powerful technologies we have today, all life forms we know of remain fundamentally limited by their biological hardware. None can live for a million years, memorize all of Wikipedia, understand all known science or enjoy spaceflight without a spacecraft. None can transform our largely lifeless cosmos into a diverse biosphere that will flourish for billions or trillions of years, enabling our Universe to finally fulfill its potential and wake up fully. All this requires life to undergo a final upgrade, to Life 3.0, which can design not only its software but also its hardware. In other words, Life 3.0 is the master of its own destiny, finally fully free from its evolutionary shackles.
The boundaries between the three stages of life are slightly fuzzy. If bacteria are Life 1.0 and humans are Life 2.0, then you might classify mice as 1.1: they can learn many things, but not enough to develop language or invent the internet. Moreover, because they lack language, what they learn gets largely lost when they die, not passed on to the next generation. Similarly, you might argue that today’s humans should count as Life 2.1: we can perform minor hardware upgrades such as implanting artificial teeth, knees and pacemakers, but nothing as dramatic as getting ten times taller or acquiring a thousand times bigger brain.
In summary, we can divide the development of life into three stages, distinguished by life’s ability to design itself:
• Life 1.0 (biological stage): evolves its hardware and software
• Life 2.0 (cultural stage): evolves its hardware, designs much of its software
• Life 3.0 (technological stage): designs its hardware and software
After 13.8 billion years of cosmic evolution, development has accelerated dramatically here on Earth: Life 1.0 arrived about 4 billion years ago, Life 2.0 (we humans) arrived about a hundred millennia ago, and many AI researchers think that Life 3.0 may arrive during the coming century, perhaps even during our lifetime, spawned by progress in AI. What will happen, and what will this mean for us? That’s the topic of this book.
From the book Life 3.0: Being Human in the Age of Artificial Intelligence by Max Tegmark, © 2017 by Max Tegmark. Published by arrangement with Alfred A. Knopf, an imprint of The Knopf Doubleday Publishing Group, a division of Penguin Random House LLC.