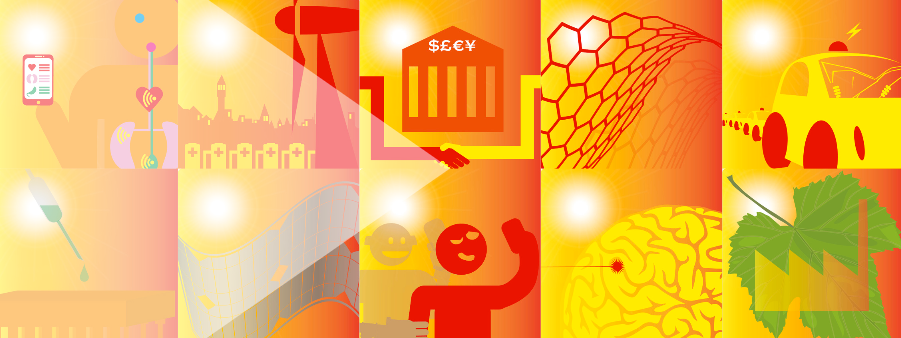
(credit: WEF)
The World Economic Forum’s annual list of this year’s breakthrough technologies, published today, includes “socially aware” openAI, grid-scale energy storage, perovskite solar cells, and other technologies with the potential to “transform industries, improve lives, and safeguard the planet.” The WEF’s specific interest is to “close gaps in investment and regulation.”
“Horizon scanning for emerging technologies is crucial to staying abreast of developments that can radically transform our world, enabling timely expert analysis in preparation for these disruptors. The global community needs to come together and agree on common principles if our society is to reap the benefits and hedge the risks of these technologies,” said Bernard Meyerson, PhD, Chief Innovation Officer of IBM and Chair of the WEF’s Meta-Council on Emerging Technologies.
The list also provides an opportunity to debate human, societal, economic or environmental risks and concerns that the technologies may pose — prior to widespread adoption.
One of the criteria used by council members during their deliberations was the likelihood that 2016 represents a tipping point in the deployment of each technology. So the list includes some technologies that have been known for a number of years, but are only now reaching a level of maturity where their impact can be meaningfully felt.
The top 10 technologies that make this year’s list are:
- Nanosensors and the Internet of Nanothings — With the Internet of Things expected to comprise 30 billion connected devices by 2020, one of the most exciting areas of focus today is now on nanosensors capable of circulating in the human body or being embedded in construction materials. They could use DNA and proteins to recognize specific chemical targets, store a few bits of information, and then report their status by changing color or emitting some other easily detectable signal.
- Next-Generation Batteries — One of the greatest obstacles holding renewable energy back is matching supply with demand, but recent advances in energy storage using sodium, aluminum, and zinc based batteries makes mini-grids feasible that can provide clean, reliable, around-the-clock energy sources to entire villages.
- The Blockchain — With venture investment related to the online currency Bitcoin exceeding $1 billion in 2015 alone, the economic and social impact of blockchain’s potential to fundamentally change the way markets and governments work is only now emerging.
- 2D Materials — Plummeting production costs mean that 2D materials like graphene are emerging in a wide range of applications, from air and water filters to new generations of wearables and batteries.
- Autonomous Vehicles — The potential of self-driving vehicles for saving lives, cutting pollution, boosting economies, and improving quality of life for the elderly and other segments of society has led to rapid deployment of key technology forerunners along the way to full autonomy.
- Organs-on-chips — Miniature models of human organs could revolutionize medical research and drug discovery by allowing researchers to see biological mechanism behaviors in ways never before possible.
- Perovskite Solar Cells — This new photovoltaic material offers three improvements over the classic silicon solar cell: it is easier to make, can be used virtually anywhere and, to date, keeps on generating power more efficiently.
- Open AI Ecosystem — Shared advances in natural language processing and social awareness algorithms, coupled with an unprecedented availability of data, will soon allow smart digital assistants to help with a vast range of tasks, from keeping track of one’s finances and health to advising on wardrobe choice.
- Optogenetics — Recent developments mean light can now be delivered deeper into brain tissue, something that could lead to better treatment for people with brain disorders.
- Systems Metabolic Engineering — Advances in synthetic biology, systems biology, and evolutionary engineering mean that the list of building block chemicals that can be manufactured better and more cheaply by using plants rather than fossil fuels is growing every year.
To compile this list, the World Economic Forum’s Meta-Council on Emerging Technologies, a panel of global experts, “drew on the collective expertise of the Forum’s communities to identify the most important recent technological trends. By doing so, the Meta-Council aims to raise awareness of their potential and contribute to closing gaps in investment, regulation and public understanding that so often thwart progress.”
You can read 10 expert views on these technologies here or download the series as a PDF.