Machine-learning algorithms are increasingly used in making important decisions about our lives — such as credit approval, medical diagnoses, and in job applications — but exactly how they work usually remains a mystery. Now Carnegie Mellon University researchers may devised an effective way to improve transparency and head off confusion or possibly legal issues.
CMU’s new Quantitative Input Influence (QII) testing tools can generate “transparency reports” that provide the relative weight of each factor in the final decision, claims Anupam Datta, associate professor of computer science and electrical and computer engineering.
Testing for discrimination
These reports could also be used proactively by an organization to see if an artificial intelligence system is working as desired, or by a regulatory agency to determine whether a decision-making system inappropriately discriminated, based on factors like race and gender.
To achieve that, the QII measures considers correlated inputs while measuring influence. For example, consider a system that assists in hiring decisions for a moving company, in which two inputs, gender and the ability to lift heavy weights, are positively correlated with each other and with hiring decisions.
Yet transparency into whether the system actually uses weightlifting ability or gender in making its decisions has substantive implications for determining if it is engaging in discrimination. In this example, the company could keep the weightlifting ability fixed, vary gender, and check whether there is a difference in the decision.
CMU researchers are careful to state in an open-access report on QII (presented at the IEEE Symposium on Security and Privacy, May 23–25, in San Jose, Calif.), that “QII does not suggest any normative definition of fairness. Instead, we view QII as a diagnostic tool to aid fine-grained fairness determinations.”
Is your AI biased?
The Ford Foundation published a controversial blog post last November stating that “while we’re lead to believe that data doesn’t lie — and therefore, that algorithms that analyze the data can’t be prejudiced — that isn’t always true. The origin of the prejudice is not necessarily embedded in the algorithm itself. Rather, it is in the models used to process massive amounts of available data and the adaptive nature of the algorithm. As an adaptive algorithm is used, it can learn societal biases it observes.
“As Professor Alvaro Bedoya, executive director of the Center on Privacy and Technology at Georgetown University, explains, ‘any algorithm worth its salt’ will learn from the external process of bias or discriminatory behavior. To illustrate this, Professor Bedoya points to a hypothetical recruitment program that uses an algorithm written to help companies screen potential hires. If the hiring managers using the program only select younger applicants, the algorithm will learn to screen out older applicants the next time around.”
Influence variables
The QII measures also quantify the joint influence of a set of inputs (such as age and income) on outcomes, and the marginal influence of each input within the set. Since a single input may be part of multiple influential sets, the average marginal influence of the input is computed using “principled game-theoretic aggregation” measures that were previously applied to measure influence in revenue division and voting.
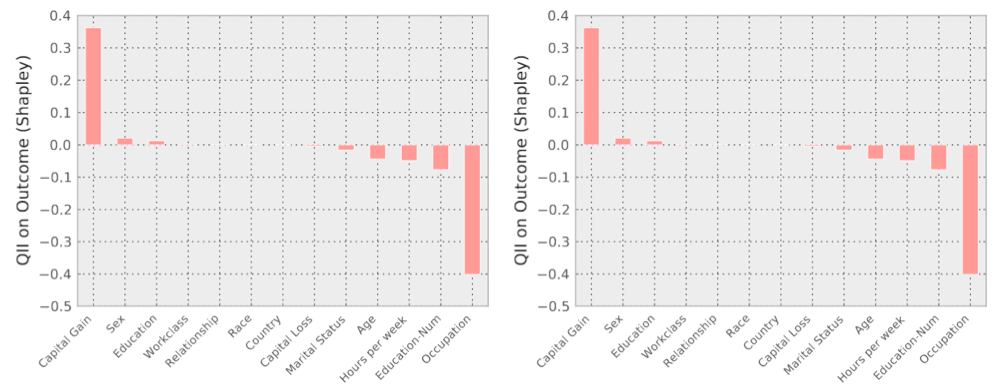
Examples of outcomes from transparency reports for two job applicants. Left: “Mr. X” is deemed to be a low income individual, an income classifier learned from the data. This result may be surprising to him: he reports high capital gains ($14k), and only 2.1% of people with capital gains higher than $10k are reported as low income. In fact, he might be led to believe that his classification may be a result of his ethnicity or country of origin. Examining his transparency report in the figure, however, we find that the most influential features that led to his negative classification were Marital Status, Relationship and Education. Right: “Mr. Y” has even higher capital gains than Mr. X. Mr. Y is a 27-year-old, with only Preschool education, and is engaged in fishing. Examination of the transparency report reveals that the most influential factor for negative classification for Mr. Y is his Occupation. Interestingly, his low level of education is not considered very important by this classifier. (credit: Anupam Datta et al./2016 P IEEE S SECUR PRIV)
“To get a sense of these influence measures, consider the U.S. presidential election,” said Yair Zick, a post-doctoral researcher in the CMU Computer Science Department. “California and Texas have influence because they have many voters, whereas Pennsylvania and Ohio have power because they are often swing states. The influence aggregation measures we employ account for both kinds of power.”
The researchers tested their approach against some standard machine-learning algorithms that they used to train decision-making systems on real data sets. They found that the QII provided better explanations than standard associative measures for a host of scenarios they considered, including sample applications for predictive policing and income prediction.
Privacy concerns
But transparency reports could also potentially compromise privacy, so in the paper, the researchers also explore the transparency-privacy tradeoff and prove that a number of useful transparency reports can be made differentially private with very little addition of noise.
QII is not yet available, but the CMU researchers are seeking collaboration with industrial partners so that they can employ QII at scale on operational machine-learning systems.
Abstract of Algorithmic Transparency via Quantitative Input Influence: Theory and Experiments with Learning Systems
Algorithmic systems that employ machine learning play an increasing role in making substantive decisions in modern society, ranging from online personalization to insurance and credit decisions to predictive policing. But their decision-making processes are often opaque—it is difficult to explain why a certain decision was made. We develop a formal foundation to improve the transparency of such decision-making systems. Specifically, we introduce a family of Quantitative Input Influence (QII) measures that capture the degree of influence of inputs on outputs of systems. These measures provide a foundation for the design of transparency reports that accompany system decisions (e.g., explaining a specific credit decision) and for testing tools useful for internal and external oversight (e.g., to detect algorithmic discrimination). Distinctively, our causal QII measures carefully account for correlated inputs while measuring influence. They support a general class of transparency queries and can, in particular, explain decisions about individuals (e.g., a loan decision) and groups (e.g., disparate impact based on gender). Finally, since single inputs may not always have high influence, the QII measures also quantify the joint influence of a set of inputs (e.g., age and income) on outcomes (e.g. loan decisions) and the marginal influence of individual inputs within such a set (e.g., income). Since a single input may be part of multiple influential sets, the average marginal influence of the input is computed using principled aggregation measures, such as the Shapley value, previously applied to measure influence in voting. Further, since transparency reports could compromise privacy, we explore the transparency-privacy tradeoff and prove that a number of useful transparency reports can be made differentially private with very little addition of noise. Our empirical validation with standard machine learning algorithms demonstrates that QII measures are a useful transparency mechanism when black box access to the learning system is available. In particular, they provide better explanations than standard associative measures for a host of scenarios that we consider. Further, we show that in the situations we consider, QII is efficiently approximable and can be made differentially private while preserving accuracy.