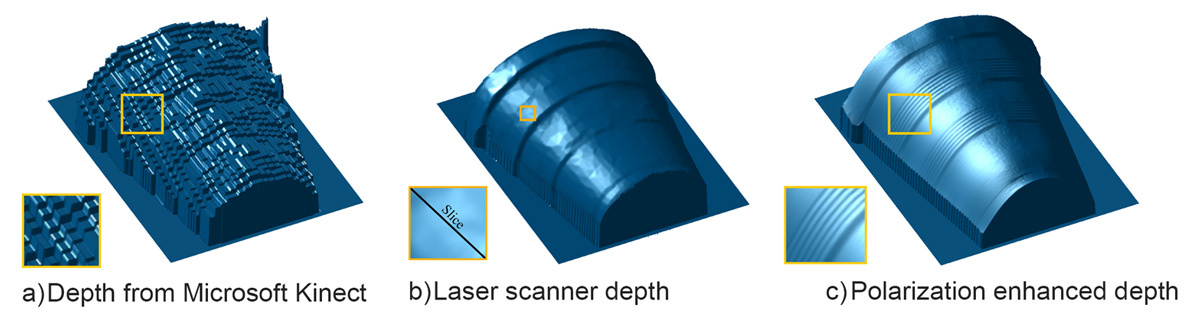
By combining the information from the Kinect depth frame in (a) with polarized photographs, MIT researchers reconstructed the 3-D surface shown in (c). Polarization cues can allow coarse depth sensors like Kinect to achieve laser scan quality (b). (credit: courtesy of the researchers)
MIT researchers have shown that by exploiting light polarization (as in polarized sunglasses) they can increase the resolution of conventional 3-D imaging devices such as the Microsoft Kinect as much as 1,000 times.
The technique could lead to high-quality 3-D cameras built into cellphones, and perhaps the ability to snap a photo of an object and then use a 3-D printer to produce a replica. Further out, the work could also help the development of driverless cars.
Headed Ramesh Raskar, associate professor of media arts and sciences in the MIT Media Lab, the researchers describe the new system, which they call Polarized 3D, in a paper they’re presenting at the International Conference on Computer Vision in December.
How polarized light works
If an electromagnetic wave can be thought of as an undulating squiggle, polarization refers to the squiggle’s orientation. It could be undulating up and down, or side to side, or somewhere in-between.
Polarization also affects the way in which light bounces off of physical objects. If light strikes an object squarely, much of it will be absorbed, but whatever reflects back will have the same mix of polarizations (horizontal and vertical) that the incoming light did. At wider angles of reflection, however, light within a certain range of polarizations is more likely to be reflected.
This is why polarized sunglasses are good at cutting out glare: Light from the sun bouncing off asphalt or water at a low angle features an unusually heavy concentration of light with a particular polarization. So the polarization of reflected light carries information about the geometry of the objects it has struck.
This relationship has been known for centuries, but it’s been hard to do anything with it, because of a fundamental ambiguity about polarized light. Light with a particular polarization, reflecting off of a surface with a particular orientation and passing through a polarizing lens is indistinguishable from light with the opposite polarization, reflecting off of a surface with the opposite orientation.
This means that for any surface in a visual scene, measurements based on polarized light offer two equally plausible hypotheses about its orientation. Canvassing all the possible combinations of either of the two orientations of every surface, in order to identify the one that makes the most sense geometrically, is a prohibitively time-consuming computation.
Polarization plus depth sensing
To resolve this ambiguity, the Media Lab researchers use coarse depth estimates provided by some other method, such as the time a light signal takes to reflect off of an object and return to its source. Even with this added information, calculating surface orientation from measurements of polarized light is complicated, but it can be done in real-time by a graphics processing unit, the type of special-purpose graphics chip found in most video game consoles.
The researchers’ experimental setup consisted of a Microsoft Kinect — which gauges depth using reflection time — with an ordinary polarizing photographic lens placed in front of its camera. In each experiment, the researchers took three photos of an object, rotating the polarizing filter each time, and their algorithms compared the light intensities of the resulting images.
On its own, at a distance of several meters, the Kinect can resolve physical features as small as a centimeter or so across. But with the addition of the polarization information, the researchers’ system could resolve features in the range of hundreds of micrometers, or one-thousandth the size.
For comparison, the researchers also imaged several of their test objects with a high-precision laser scanner, which requires that the object be inserted into the scanner bed. Polarized 3D still offered the higher resolution.
Uses in cameras and self-driving cars
A mechanically rotated polarization filter would probably be impractical in a cellphone camera, but grids of tiny polarization filters that can overlay individual pixels in a light sensor are commercially available. Capturing three pixels’ worth of light for each image pixel would reduce a cellphone camera’s resolution, but no more than the color filters that existing cameras already use.
The new paper also offers the tantalizing prospect that polarization systems could aid the development of self-driving cars. Today’s experimental self-driving cars are, in fact, highly reliable under normal illumination conditions, but their vision algorithms go haywire in rain, snow, or fog.
That’s because water particles in the air scatter light in unpredictable ways, making it much harder to interpret. The MIT researchers show that in some very simple test cases their system can exploit information contained in interfering waves of light to handle scattering.
Abstract of Polarized 3D: High-Quality Depth Sensing with Polarization Cues
Coarse depth maps can be enhanced by using the shape information from polarization cues. We propose a framework to combine surface normals from polarization (hereafter polarization normals) with an aligned depth map. Polarization normals have not been used for depth enhancement before. This is because polarization normals suffer from physics-based artifacts, such as azimuthal ambiguity, refractive distortion and fronto-parallel signal degradation. We propose a framework to overcome these key challenges, allowing the benefits of polarization to be used to enhance depth maps. Our results demonstrate improvement with respect to state-of-the-art 3D reconstruction techniques